top of page
Contextualized Poverty Targeting
Exploring contextualized targeting methods through multimodal spatial data and ML in a data-deficient city

Congo

Congo
1/1
Project Description
AI/ML models are predominantly developed in countries with georeferenced national surveys. “Contextualized Poverty Targeting Through Multimodal Spatial Data and Machine Learning in Brazzaville, Congo” demonstrates that household-level targeting can be achieved in a data deficient city without a gold standard survey. Using the case of Brazzaville, this study integrates intuitive images, social media, points of interest, connectivity, and administrative data to predict multidimensional poverty at the household level. The simulation in this study shows that a spatially augmented targeting method not only reduces targeting errors but also decreases the poverty ratio, gap, and severity.
Abstract
Enhancing targeting accuracy in social welfare programs fosters equitable urban development. Advancements in this field harness georeferenced data and leverage AI/machine learning (ML) techniques to predict poverty and allocate aid. However, these models are predominantly developed in areas with georeferenced national surveys and are intended for geographic targeting. We demonstrate that micro, household-level targeting can be achieved in understudied cities lacking ground truth data. Using the case of Brazzaville in Congo, we integrate intuitive images, social media, points of interest, connectivity, and administrative data to predict multidimensional poverty at the household level. The simulation in this study demonstrates that ML-based targeting not only reduces targeting errors but also decreases the poverty ratio, gap, and severity. Our spatially augmented model, surpassing status quo mechanisms, can promote inclusive social welfare programs at hyper-granular levels in urban areas. Given the rapid urbanization in developing countries, this study’s data collection and fine-tuning process is relevant and applicable to other data-sparse contexts.
FOOD INSECURITY IN CONGO PRE-COVID
FOOD INSECURITY IN CONGO DURING COVID
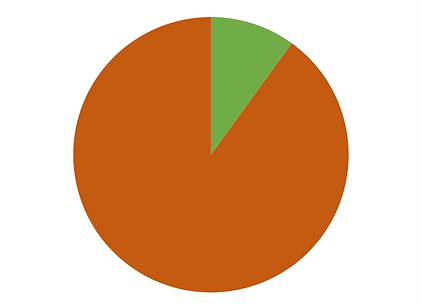
FOOD
SECURITY
90%
FOOD
INSECURITY
10%

FOOD
INSECURITY
30%
FOOD
SECURITY
70%
Artificial intelligence (AI) and machine learning (ML) techniques can generate unbiased geographical data and identify areas of extreme poverty, which policymakers can adopt to prioritize development aid allocations. This innovative method can help develop scalable algorithms to inform humanitarian responses to Africa's food crisis. In the Republic of the Congo (RoC), one out of two people live below poverty, and the pre-COVID level of food insecurity of 10% has surged to 30% during the pandemic. Thus far, governments have responded to this crisis by doubling social protection programs. However, the current targeting methods do not provide enough information on eligible communities and are too slow to mitigate transitory shocks. This issue highlights the need for a systemic, fine-grained, and rapid geographic targeting technique.
..................................................................................................................................................................................
Multimodal Approach in Brazzaville, Congo
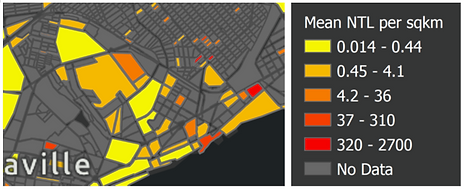
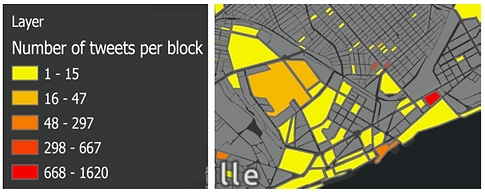
TWITTER COUNT
NIGHTTIME LIGHTS

PARTNERS ....................................................

Recipient of Microsoft
AI for Humanitarian Action Project Initiatives
$25,000 Azure Grant
PARTNERS ....................................................

PARTNERS
..........................................................
PARTNERS ....................................................
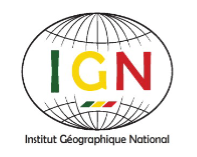

Image by Annie Spratt

Image by Annie Spratt
1/1
Dr. Jung’s research is expected to have high global impact by offering enhanced data for key development actors to reach people and regions with extreme poverty. This project's outputs, such as high-resolution poverty maps, will be shared with the WFP and the Ministry of Social Affairs to scale up social protection programs. These maps can be updated as new data becomes available to decision-makers in near real time. This work could potentially address misinformation and current criticisms of AI that miss out on the bottom billion, thereby addressing diversity, equity, and inclusion.
RESEARCH TEAM .................................................................
The team consists of computer/data scientists
and social scientists:
-
Woojin Jung (PI) - Rutgers, School of Social Work
-
Dmitris Metaxas - Rutgers, Computer Science
-
Quentin Stoeffler - University of Bordeaux, Economics
-
Saeed Ghadimi - University of Waterloo, AI Institute
-
Dimitrios Ntarlagiannis - Rutgers, Earth and Env. Sciences
-
Arunesh Sinha - Rutgers, Mgmt Science and Information Systems
-
Min Xu - Rutgers, Statistics
-
Tawfiq Ammari - Rutgers, Communication and Information
-
Andrew Kim - Rutgers, School of Social Work
RESEARCH TEAM .................................................................
-
Maryam Hosseini - MIT, Urban Studies and Planning
-
Melissa Sartorius - Rutgers, School of Social Work
-
Sajedeh Goudarzi - Rutgers, Global Urban Studies
-
William Benjamin - Allstate Corp., Data Science
-
Rofaida Benotsmane - Istanbul Technical Univ., Economics
-
Yuxiao Lu - Singapore Management Univ., Computer Science
-
Vatsal Shah - Rutgers, Computer Science, Statistics
-
EJ Knopf - Rutgers, School of Social Work
-
Jamie Cramer - Rutgers, School of Social Work
The research team represents diversity across disciplines, countries/continents (Africa, Asia, US), languages, and cultures. Our study can contribute to broadening the reach of social protection programs by identifying vulnerable and poor populations in the Republic of the Congo and other countries where there is very limited, georeferenced wealth data.
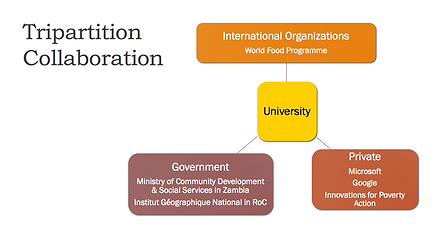
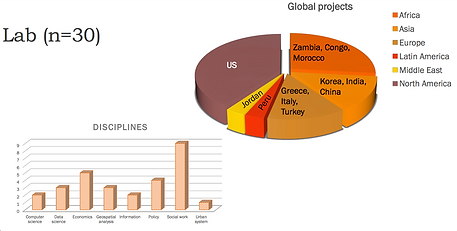
This current project in the Republic of the Congo was recently featured in 'Focus on Faculty' on Rutgers University's Office of Research website and social media outlets: .
An impact report is also featured in the Rutgers Global Health Institute's 2021-2022 Annual Report.
bottom of page