top of page
The Last Mile in Remote Sensing Poverty Prediction
Utilizing a Multimodal Model to achieve near-perfect poverty prediction in difficult-to-predict regions

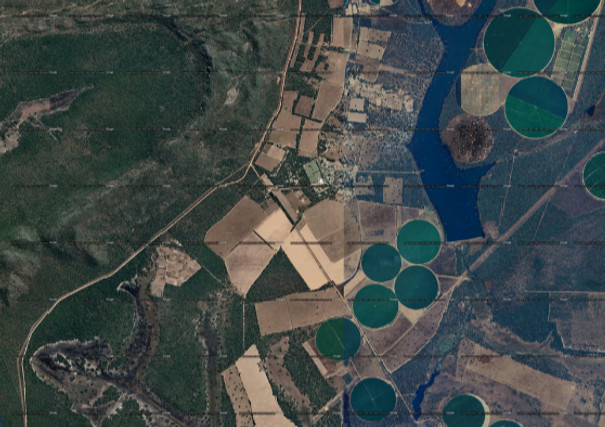
Abstract
A prominent approach in remote sensing poverty prediction uses deep learning satellite imagery with daytime and nighttime light (NTL). While results yield acceptable average predictions, certain areas consistently perform poorly. For high-stakes aid allocation decisions, however, achieving near-perfect predictions across all regions is crucial. To address this, we apply explainability techniques to evaluate vision models, enhance predictions with multimodal architectures, and investigate challenging cases. Our diagnostic analysis shows that fine-tuning with NTL tends to fail in areas where NTL poorly reflects wealth. Integrated Gradients and Guided Grad-CAM techniques also reveal that VGG, ResNet, and ViT architectures tend to focus on brightly lit features, such as roads and buildings. To complement vision models, a multimodal model integrates non-visual features. Metrics such as Twitter activity, distance from residential roads, and internet speed are especially predictive. Among ten tested architectures, the Stack Ensemble Late Stage model, combining pre-trained weights, multimodal data, and NTL transfer learning, achieves the best performance with high accuracy and low error variance. Qualitative analysis of difficult-to predict regions highlights the challenges of modeling wealth due to inherent aleatoric uncertainty. Two core issues, spatial inequality in small areas and the presence of rare features, underscore the need for principled cluster segmentation.
Citation
Jung, W., Sinha, A., Kim, A. H., Shah, V., Lu, Y., Ammari, T., & Lee, L. (2025, In press). The last mile in remote sensing poverty prediction. Association for Computing Machinery (ACM) Journal on Computing and Sustainable Societies.
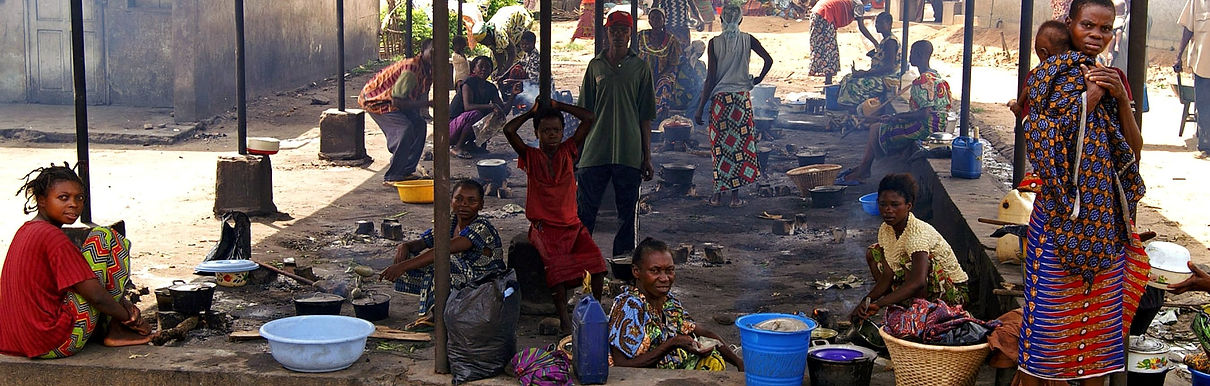
NEWS
Dr. Woojin Jung has been invited to present this study at the Association for Computing Machinery (ACM) Conference on Computing and Sustainable Societies (COMPASS) at the University of Toronto in Toronto, Canada, on July 22-25, 2025.
bottom of page